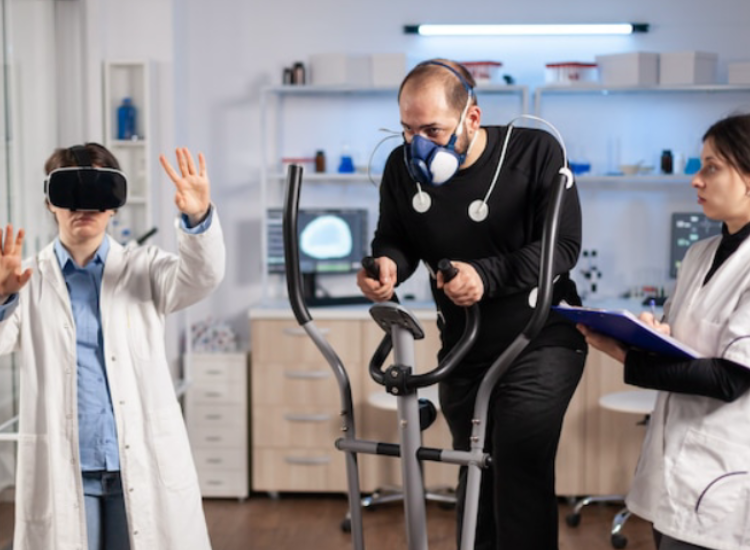
Utilizing IoT Sensors and Wearable Devices with Machine Learning for Real-time Monitoring and Analysis of Patient Vital Signs
IoT sensors and wearable devices can collect data on a patient's vital signs such as heart rate, blood pressure, and oxygen saturation in real-time. Machine learning models, including recurrent neural networks (RNNs) and convolutional neural networks (CNNs), are used to analyze this data for abnormalities and alert healthcare providers when necessary.
The convergence of Internet of Things (IoT) technologies and machine learning within the healthcare sector is heralding a new era in patient monitoring and disease prevention. Recent studies have demonstrated how IoT sensors and wearable devices, when coupled with sophisticated machine learning algorithms, are transforming the landscape of health monitoring, offering unprecedented opportunities for early disease detection and personalized healthcare management.
IoT-enabled health monitoring systems are at the forefront of this revolution, leveraging a multitude of sensors integrated into wearable devices to collect vital health data in real-time. These devices monitor an array of patient vital signs, including heart rate, blood pressure, and oxygen saturation, providing a continuous stream of health data. This wealth of information is then transmitted to centralized servers, where advanced machine learning models, including recurrent neural networks (RNNs) and convolutional neural networks (CNNs), analyze the data to detect any abnormalities or signs of emerging health issues.
The real power of these technologies lies in their ability to identify potential health problems before they become critical, allowing for timely intervention by healthcare providers. Machine learning models, trained on vast datasets, can recognize subtle deviations from normal health patterns, flagging early indicators of diseases and health anomalies even before symptoms become apparent to the patient.
Furthermore, these systems do not just stop at disease detection. They play a crucial role in empowering patients with personalized health insights and actionable recommendations. Based on the analysis of collected data, wearable devices can offer personalized advice on lifestyle adjustments, sleep optimization, and dietary changes to promote overall wellness. This approach fosters a proactive rather than reactive healthcare model, encouraging individuals to take an active role in managing their health.
Despite the promising advancements, the integration of IoT and machine learning in healthcare is not without challenges. Issues such as data privacy, security concerns, and the need for scalable solutions remain paramount. Ensuring the confidentiality and integrity of patient data while developing systems that can be widely adopted across diverse healthcare settings is critical to the success of these technologies.
In essence, the synergy between IoT sensors, wearable devices, and machine learning is setting the stage for a healthcare paradigm shift. By enabling real-time monitoring, early detection of health issues, and personalized health management, these technologies are not just enhancing patient care but are also paving the way for a future where preventive healthcare takes precedence, ultimately improving quality of life and reducing healthcare costs.