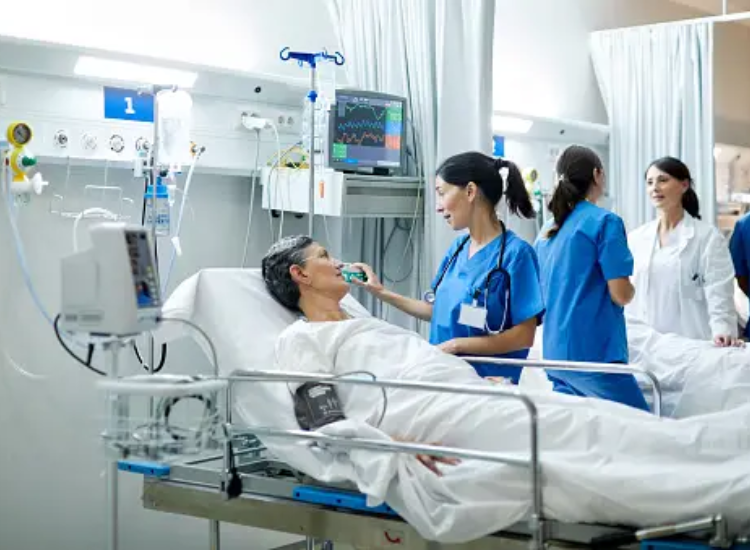
Unlocking the Power of Data in Predicting Hospital Length of Stay
In the realm of healthcare, efficiently managing hospital length of stay (LOS) for patients is not just about cutting costs—it's about optimizing care, improving patient satisfaction, and enhancing hospital operations. Two recent studies have shed light on innovative approaches to predict LOS, leveraging both structured and unstructured data through advanced machine learning techniques. Here's how these studies are paving the way for smarter healthcare
Harnessing Unstructured Data for Precision
A study conducted by Jan Chrusciel and colleagues, published in BMC Medical Informatics and Decision Making, explored the potential of unstructured electronic health records (EHRs) to improve LOS predictions. Traditional models have relied on structured data like age, gender, and ICD diagnoses. However, this study compared these conventional models against a new model that also included clinical signs from unstructured text data.
The findings were enlightening. The model incorporating unstructured data achieved slightly higher accuracy in predicting LOS compared to the structured data model. This suggests that valuable clinical insights, often buried within the narrative sections of EHRs, can play a crucial role in forecasting hospital stays. By extracting clinical information from text notes using a word-embedding algorithm, hospitals can potentially refine their LOS prediction models, leading to better resource allocation and patient care.
Addressing Data Challenges for Enhanced Predictions
Another pivotal study by Amin Naemi and colleagues, also featured in BMC Medical Informatics and Decision Making, tackled key challenges in LOS prediction, such as missing values and data skewness. Recognizing that incomplete records and skewed LOS data can significantly impact model performance, the researchers proposed a comprehensive method involving multivariate imputation techniques and proper resampling strategies.
Their approach led to significant improvements in the accuracy of machine learning models predicting LOS. By addressing these data challenges head-on, the study not only enhanced the prediction models' performance but also underscored the importance of considering the complexity and nuances of healthcare data in developing predictive analytics.
Future Directions and Implications
These studies highlight the untapped potential of both unstructured EHR data and sophisticated data preprocessing techniques in improving LOS predictions. As healthcare continues to evolve towards data-driven decision-making, these findings offer valuable insights for hospital administrators, clinicians, and data scientists alike.
The ability to accurately predict LOS has profound implications for patient care planning, hospital resource management, and overall operational efficiency. Future research could explore the integration of diverse data sources, the application of deep learning models, and the development of real-time prediction systems to further enhance the accuracy and utility of LOS forecasts.
In conclusion, the innovative use of unstructured data and the strategic handling of common data challenges represent significant strides in the quest for better hospital LOS predictions. As the healthcare industry embraces these advanced analytics approaches, the prospects for improved patient outcomes and more efficient hospital operations look increasingly promising.
Monitoring the progression of chronic diseases like Parkinson's or Alzheimer's by predicting changes in patient symptoms over time